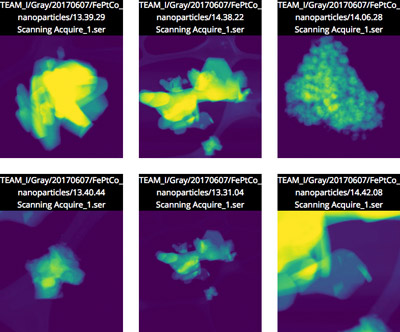
As scientific datasets increase in both size and complexity, the ability to label, filter and search this deluge of information has become a laborious, time-consuming and sometimes impossible task, without the help of automated tools.
With this in mind, a team of researchers from the Berkeley Lab and UC Berkeley are developing innovative machine learning tools to pull contextual information from scientific datasets and automatically generate metadata tags for each file. Scientists can then search these files via a web-based search engine for scientific data, called Science Search, that the Berkeley team is building.
As a proof-of-concept, the team is working with staff at Berkeley Lab’s Molecular Foundry, to demonstrate the concepts of Science Search on the images captured by the microscopes at the Foundry’s National Center for Electron Microscopy (NCEM). A beta version of the platform has been made available to Foundry researchers.
Today, search engines are ubiquitously used to find information on the Internet but searching science data presents a different set of challenges. For example, Google’s algorithm relies on more than 200 clues to achieve an effective search. These clues can come in the form of key words on a webpage, metadata in images or audience feedback from billions of people when they click on the information they are looking for. In contrast, scientific data comes in many forms that are radically different than an average web page, requires context that is specific to the science and often also lacks the metadata to provide context that is required for effective searches.
At National User Facilities like the Molecular Foundry, researchers from all over the world apply for time and then travel to Berkeley to use extremely specialized instruments free of charge. Current cameras on microscopes at the Foundry can collect up to a terabyte of data in under 10 minutes. Users then need to manually sift through this data to find quality images with “good resolution” and save that information on a secure shared file system, like Dropbox, or on an external hard drive that they eventually take home with them to analyze.
To address the metadata issue, the Berkeley Lab team uses machine-learning techniques to mine the “science ecosystem”—including instrument timestamps, facility user logs, scientific proposals, publications and file system structures—for contextual information. The collective information from these sources including timestamp of the experiment, notes about the resolution and filter used and the user’s request for time, all provides critical contextual information. The Berkeley lab team has put together an innovative software stack that uses machine-learning techniques including natural language processing pull contextual keywords about the scientific experiment and automatically create metadata tags for the data.
To facilitate an effective search for users based on available information, the team’s search interface provides a query mechanism for available files, proposals and papers that the Berkeley-developed machine-learning tools have parsed and extracted tags from. Each listed search result item represents a summary of that data, with a more detailed secondary view available, including information on tags that matched this item. The team is currently exploring how to best incorporate user feedback to improve the models and tags.