Scientific Achievement
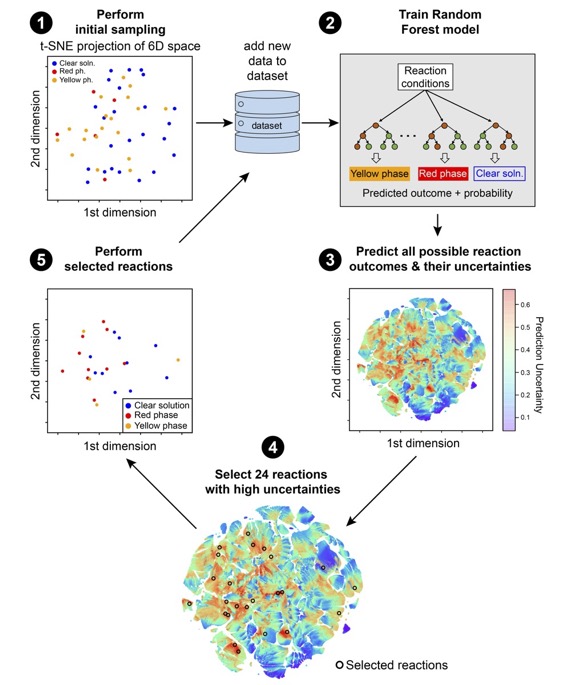
Foundry staff and users coupled a robotic workflow with active learning to discover, control, and understand the formation of metal halide perovskites (MHPs) with different dimensionalities (1D and 2D).
Significance and Impact
This is the first report of using active learning and high-throughput synthesis to control the dimensionality of MHPs. This data-driven approach will be valuable for controlling the crystallization of a range of materials with desirable optoelectronic properties.
Research Details
- The researchers developed an active learning model to train machine learning classification models to predict the dimensionalities of phases formed in the morpholinium lead iodide (morph-Pb-I) system.
- They established and validated a robust stopping criterion to determine when an active-learning experiment should be stopped and uncovered the reaction parameters that have the most significant effects on dimensionality control.
- The team coupled the model insights with density functional theory calculations to propose a reaction mechanism that describes the selective formation of 1D or 2D morpholinium lead iodide structures.